Analytics FC’s Alex Stewart looks at an important update to the goal scoring metrics in our TransferLab data-scouting tool since the launch the v3 version of the platform
TransferLab has undergone an update for its shots metrics (expected goals and finishing). We have now split shots with feet and headed shots, while also creating a combined metric to account for both. These metrics are available for both Expected Goals and also for our GDA-powered ‘finishing’ value. If you want a quick overview of our market leading Goal Difference Added algorithm click on the video below:
Why is this change important?
The update to our shot metrics gives even more flexibility when it comes to creating player profiles, already one of TransferLab’s most powerful tools. Users are now able to choose from the following metrics when creating profiles:
Expected Goals (shots – open play), Expected Goals (headers – open play), and Expected Goals (combined); and Finishing (shots – open play), Finishing (headers – open play), Finishing (headers – set pieces), and Finishing (combined).
So, how does this split help us? Well, the first thing to recognise is that an overview of a player’s expected goals (combine all shots, both feet and head) can sometimes be misleading. Take the example below of Dominic Calvert-Lewin. Assessed against Tier 1 strikers, his combined expected goals is a very healthy 86% percentile, meaning he is in the top 14% for all strikers at this level.
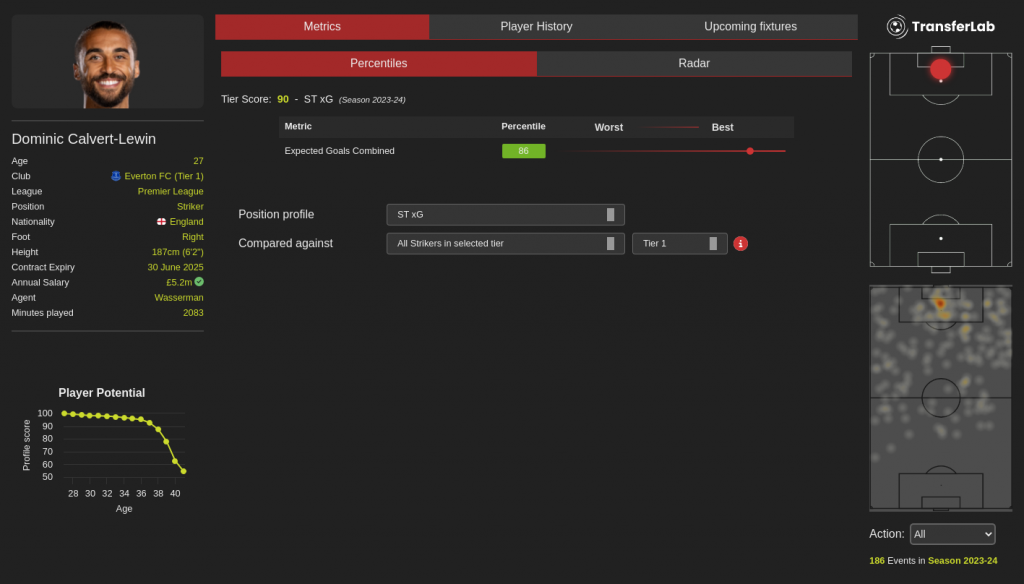
Splitting that combined xG out, though, gives us far greater clarity around the player’s output. Calvert-Lewin is producing elite levels of expected goals from headed shots in open play, in the top 3% of all Tier 1 strikers, but his expected goals from open play non-headed shots is far lower, in the 63rd percentile. While obviously a top-tier all-round striker, Calvert-Lewin’s output is pretty starkly split between headed and non-headed shots, and that needs to be accounted for when assessing the player.
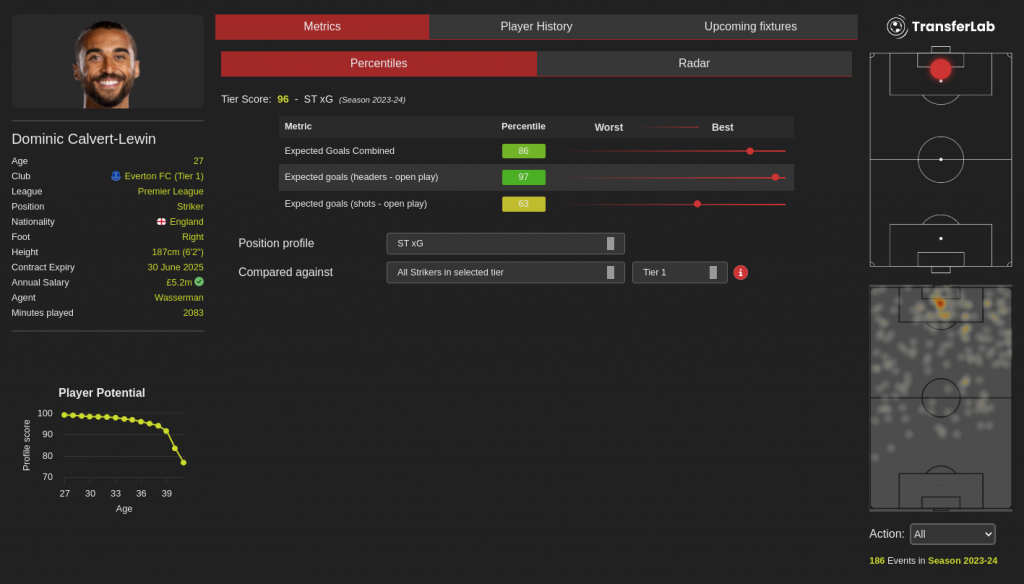
It’s easier to find the inverse, players who excel on the ground but not in the air, but a particularly clear example is former FC Midtjylland, now Benfica striker Casper Tengstedt. Among Tier 1 strikers his overall expected goals is a very creditable top 23%, but when split out, you can see that his elite output from non-headed shots (95th percentile) massively eclipses his output in the air (22nd percentile).
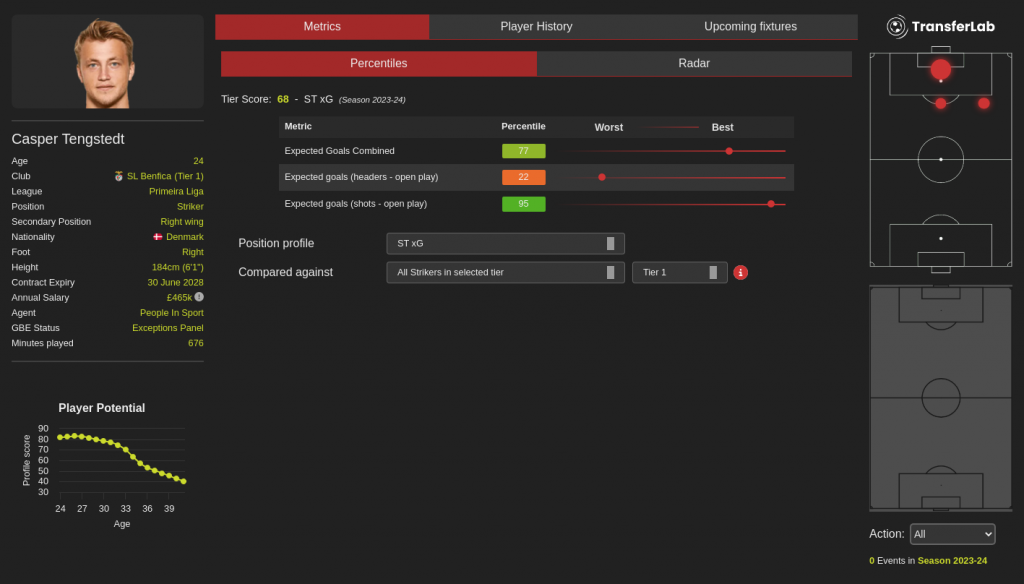
This split also means that we can look in greater detail for specific types of player who suit specific approaches, or requires a specific profile of player for its game model. By splitting xG into shots from feet and shots from headers, we can find different styles of player, ones who thrive off through balls or cutbacks, for example, versus ones who score well from crosses.
The fox in the box
So, let’s create a profile for both kinds of striker. Keeping it simple, our ‘fox-in-the-box’ striker will be assessed on expected goals from open play (foot) shots, finishing (shots – open play), touches in box, forward passes received, goal importance, shot conversion rate, and shots per 90 (a quantity, not quality, metric). Obviously, a fuller profile could be created using more of TransferLab’s quality or quantity metrics, but for now we shall hone in on the most immediately relevant for this profile of player.
Assessing players among Tier 1 clubs who have played 500 or more minutes this season just finished, we can see the most effective predatory strikers (of course, like Harry Kane, they may well have copious other qualities).
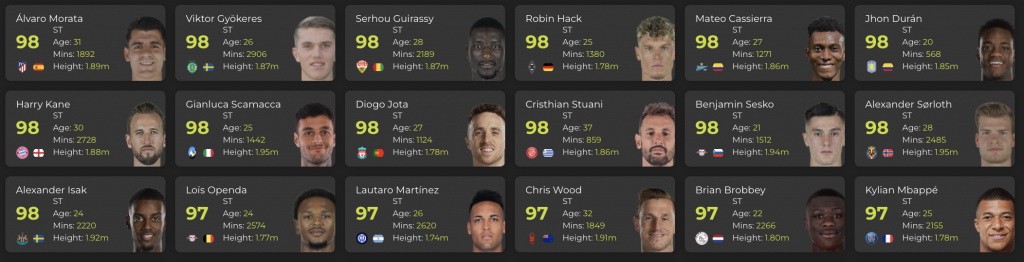
This profile shows that Viktor Gyokeres has been deserving of the attention received since his move to Sporting: he is in the top 10% of strikers in Liga NOS for each selected metric save shots per 90 and has yet to reach his peak.
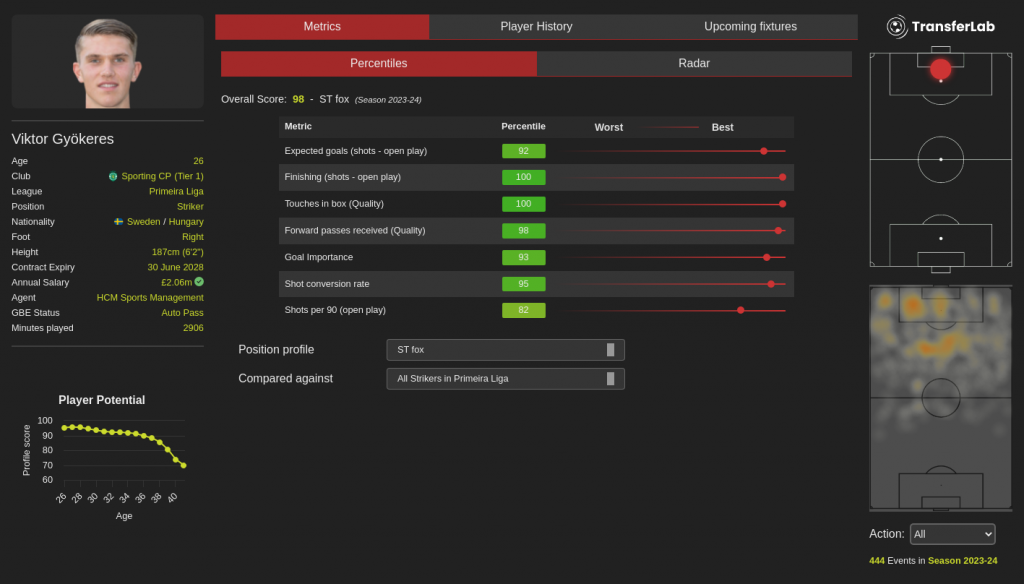
Meanwhile, Serhou Guirassy, who when compared with other Bundesliga strikers, shows elite levels across the board. It’s no surprise that he tied Kane last season for non-penalty goals per 90, while also coming third in the Bundesliga for non-penalty expected goals per 90.
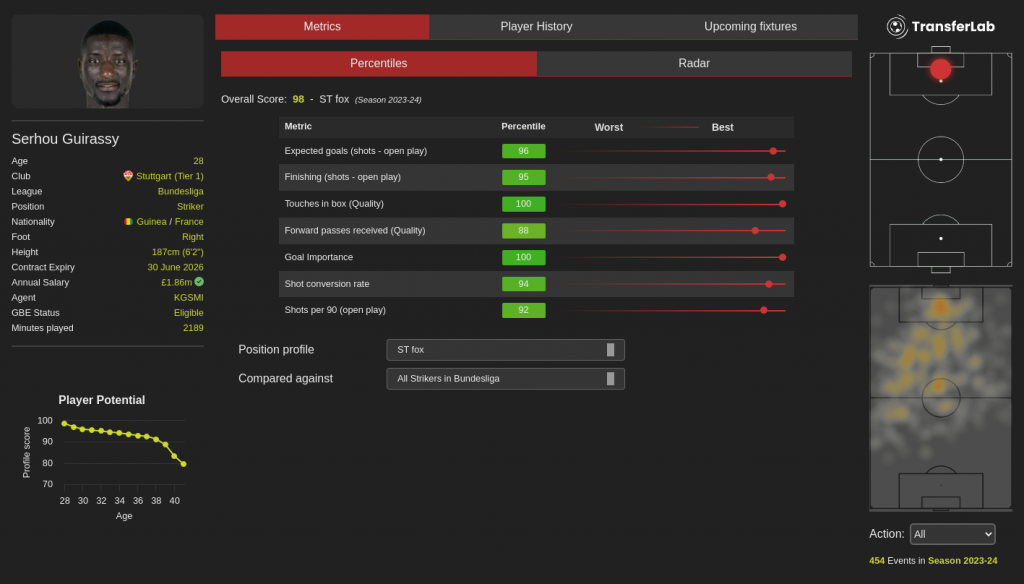
The target man
Moving now to more of a “target man” profile, focusing on expected goals and finishing from open play headers, as well as a number of other heading metrics. Again, we have looked at strikers with over 500 minutes played for Tier 1 clubs and, again, we have selected a much reduced number of metrics.
As we can see, the list of players is markedly different, focusing much more on tall, strong players who can win headers, generate good opportunities from crosses, and win fouls. Diogo Jota and Benjamin Sesko are the only players on both lists.
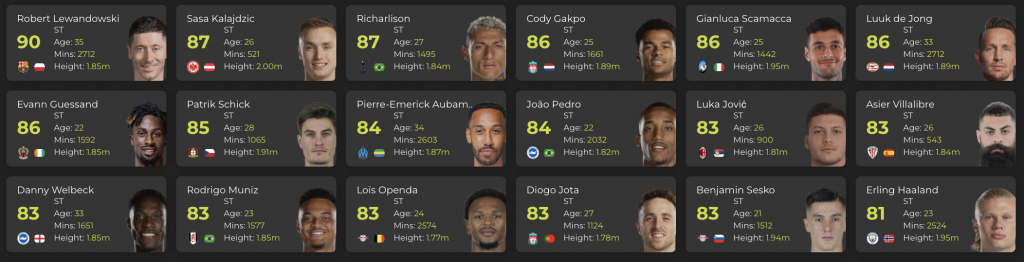
Stuttgart’s Sasa Kalajdzc is a fine example of target striker, who is in the top 1% of strikers in the Bundesliga for finishing from headers and top 25% for xG from headers (open play).
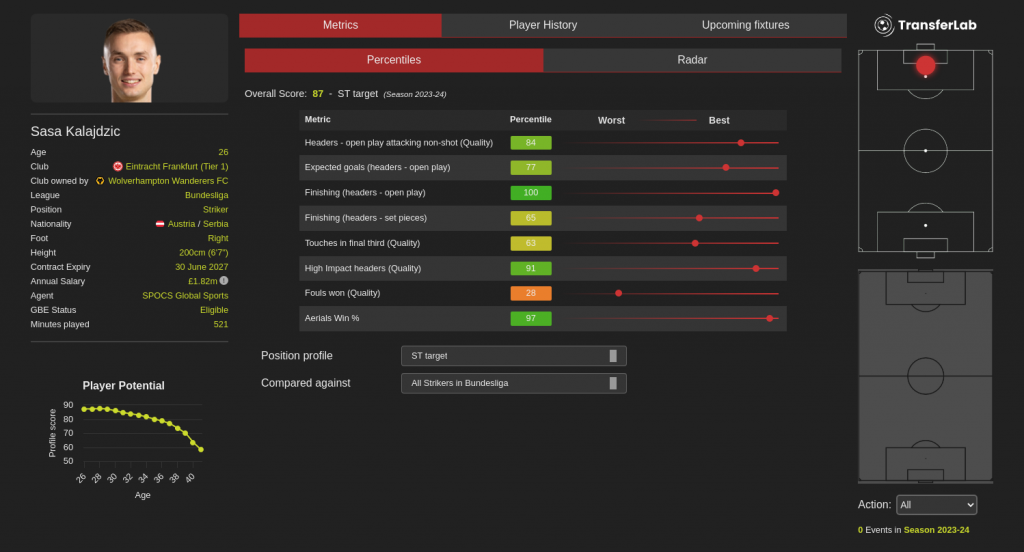
Nice’s Evann Guessand, an excellent pressing striker, is also a top 11% striker for both open play expected goals and finishing from headers; he also wins a lot of fouls and this profile suggests he could excel as a target striker for a high pressing side.
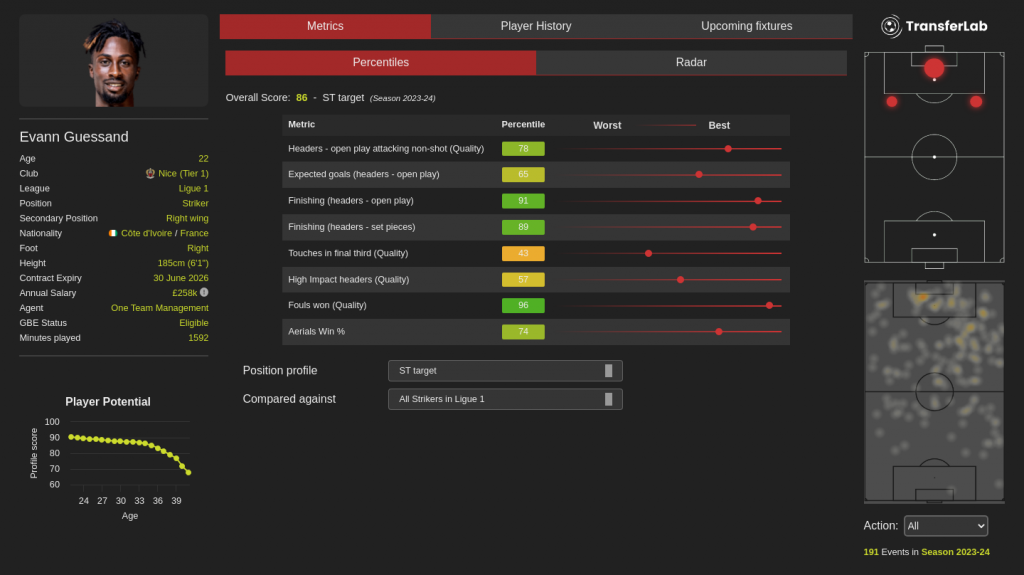
Finishing school
This new metrics also afford us the opportunity to assess players who may generate copious expected goals, but whose finishing lets them down, or vice versa, players who finish superbly (or score unlikely goals) but for one reason or another (for example, lack of opportunities generated from team-mates, strong defensive opposition, or the effect of game state on substitutes) may fail to generate significant xG numbers.
Consider, for example, the Premier League’s top 18 players ranked by xG per 90 for open play shots with feet. The usual suspects are present: Haaland, Muniz, Nunez, and so on.
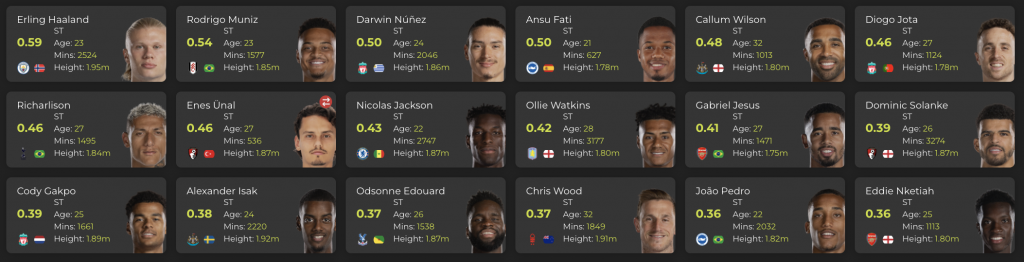
Then consider the top 18 ranked by the GDA contribution of their Finishing (shots – open play). These are the players whose finishing quality with feet, rather than the expected goals generated, stand out, either by not missing many high value chances, or scoring from a few very low value shots (especially relevant for midfielders or defenders with low cumulative xG and few shots per 90).
Callum Wilson, Diogo Jota, Richarlison, and Chris Wood are the only three players in both lists. Noted xG over-performer Son Heung-min also scores highly for his finishing, unsurprisingly.
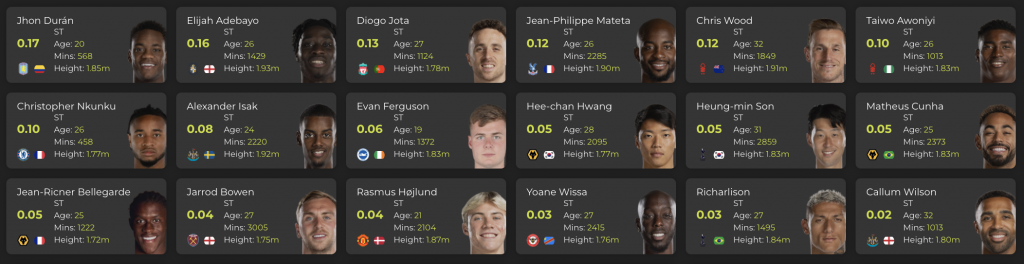
Adding in headed finishes from open play and set pieces to get a combined finishing quality, we can see that Luton Town’s Elijah Adebayo contributed most per 90 to his team’s Goal Difference Added of all Premier League strikers last season, while Aston Villa’s Jhon Durán and Liverpool’s Diogo Jota complete the top three.
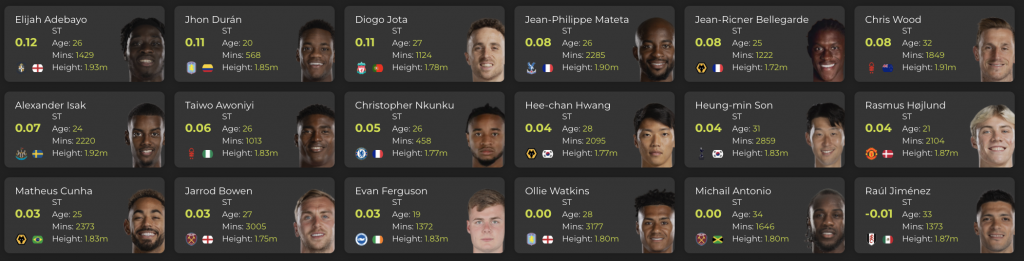
Of these, only Jota also weighs in with significant expected goals, a reflection of the ability to generate good shooting opportunities. It, of course, also dependant, in part at least, on their team’s ability to put them in a position to generate such chances. This means that the finishers in our list who do not generate high levels of xG per 90 could have been hampered by the chances created for them by their teams, or do surprisingly well finishing their own low xG chances.
More metrics, more sophistication
As we have seen, splitting these metrics, expected goals and finishing, into constituent shot types allows us to be more specific in our player profile creation, which means that teams can hone in even more on the kind of players that suit their game model.
If you want to learn more about our TransferLab platform contact us here, or book a free trial of the by clicking here.
Header image copyright IMAGO / Propaganda Photo / David Rawcliffe